来源:llm/sglang
SGLang: 一个结构化生成语言#
此 README 包含运行 SGLang 演示的说明。SGLang 是一个开源库,用于快速且富有表现力的 LLM 推理和服务,吞吐量可达 5 倍。
先决条件#
安装最新版本的 SkyPilot 并检查您的云凭证设置
pip install "skypilot-nightly[all]"
sky check
使用 SkyServe 通过 SGLang 提供视觉-语言模型 LLaVA 以处理更多流量#
创建包含
service
部分的SkyServe 服务 YAML
service:
# Specifying the path to the endpoint to check the readiness of the service.
readiness_probe: /health
# How many replicas to manage.
replicas: 2
完整的服务 YAML 文件在此处:llava.yaml。
使用 SkyServe CLI 启动服务
sky serve up -n sglang-llava llava.yaml
使用
sky serve status
检查服务的状态
sky serve status sglang-llava
您应该会看到与以下类似的输出
Services
NAME VERSION UPTIME STATUS REPLICAS ENDPOINT
sglang-llava 1 8m 16s READY 2/2 34.32.43.41:30001
Service Replicas
SERVICE_NAME ID VERSION IP LAUNCHED RESOURCES STATUS REGION
sglang-llava 1 1 34.85.154.76 16 mins ago 1x GCP({'L4': 1}) READY us-east4
sglang-llava 2 1 34.145.195.253 16 mins ago 1x GCP({'L4': 1}) READY us-east4
检查服务的端点
ENDPOINT=$(sky serve status --endpoint sglang-llava)
一旦状态显示为
READY
,您就可以使用该端点与模型进行文本和图像输入交互
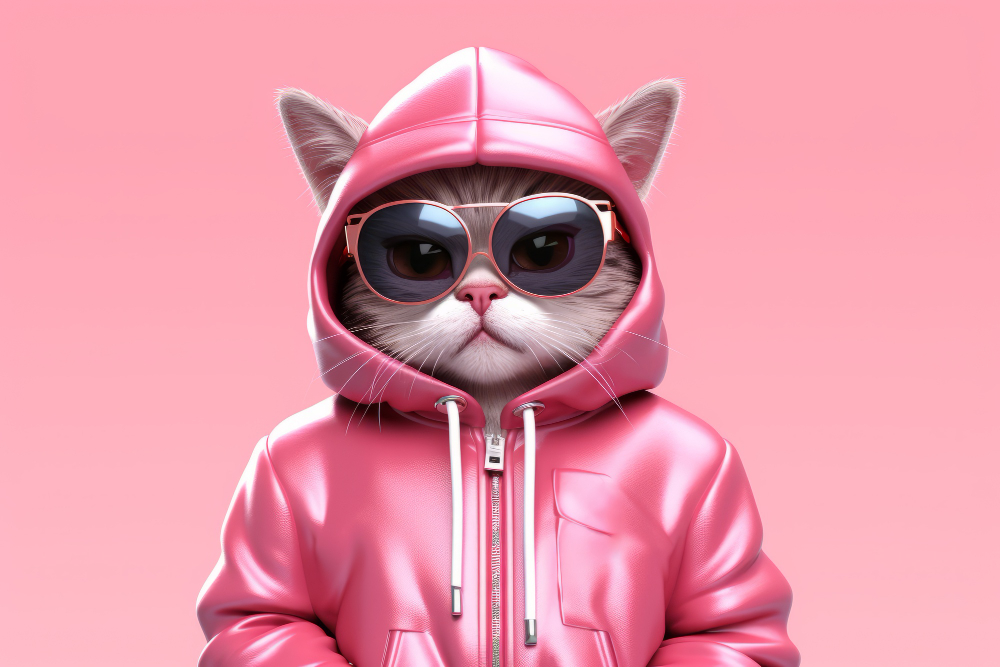
curl $ENDPOINT/v1/chat/completions \
-H "Content-Type: application/json" \
-d '{
"model": "liuhaotian/llava-v1.6-vicuna-7b",
"messages": [
{
"role": "user",
"content": [
{"type": "text", "text": "Describe this image"},
{
"type": "image_url",
"image_url": {
"url": "https://raw.githubusercontent.com/sgl-project/sglang/main/examples/frontend_language/quick_start/images/cat.jpeg"
}
}
]
}
]
}'
您应该会收到与以下类似的响应
{
"id": "b044d5f637694d3bba30a2d784441c6c",
"object": "chat.completion",
"created": 1707565348,
"model": "liuhaotian/llava-v1.6-vicuna-7b",
"choices": [{
"index": 0,
"message": {
"role": "assistant",
"content": " This is an image of a cute, anthropomorphized cat character."
},
"finish_reason": null
}],
"usage": {
"prompt_tokens": 2188,
"total_tokens": 2204,
"completion_tokens": 16
}
}
使用 SkyServe 通过 SGLang 提供 Llama-2 以处理更多流量#
过程与提供 LLaVA 相同,但模型路径更改为 Llama-2。以下为参考示例命令。
使用 SkyServe CLI 启动服务
sky serve up -n sglang-llama2 llama2.yaml --env HF_TOKEN=<your-huggingface-token>
完整的服务 YAML 文件在此处:llama2.yaml。
使用
sky serve status
检查服务的状态
sky serve status sglang-llama2
您应该会看到与以下类似的输出
Services
NAME VERSION UPTIME STATUS REPLICAS ENDPOINT
sglang-llama2 1 8m 16s READY 2/2 34.32.43.41:30001
Service Replicas
SERVICE_NAME ID VERSION IP LAUNCHED RESOURCES STATUS REGION
sglang-llama2 1 1 34.85.154.76 16 mins ago 1x GCP({'L4': 1}) READY us-east4
sglang-llama2 2 1 34.145.195.253 16 mins ago 1x GCP({'L4': 1}) READY us-east4
检查服务的端点
ENDPOINT=$(sky serve status --endpoint sglang-llama2)
一旦状态显示为
READY
,您就可以使用该端点与模型进行交互
curl $ENDPOINT/v1/chat/completions \
-H "Content-Type: application/json" \
-d '{
"model": "meta-llama/Llama-2-7b-chat-hf",
"messages": [
{
"role": "system",
"content": "You are a helpful assistant."
},
{
"role": "user",
"content": "Who are you?"
}
]
}'
您应该会收到与以下类似的响应
{
"id": "cmpl-879a58992d704caf80771b4651ff8cb6",
"object": "chat.completion",
"created": 1692650569,
"model": "meta-llama/Llama-2-7b-chat-hf",
"choices": [{
"index": 0,
"message": {
"role": "assistant",
"content": " Hello! I'm just an AI assistant, here to help you"
},
"finish_reason": "length"
}],
"usage": {
"prompt_tokens": 31,
"total_tokens": 47,
"completion_tokens": 16
}
}
使用 SGLang 提供 Llama-4#
关于如何在 SGLang 上提供 Llama 4(单节点和多节点)的社区教程,请参阅 使用 SkyPilot 和 SGLang 在 Nebius AI Cloud 上提供 Llama 4 模型。
包含的文件#
llama2.yaml
service:
# Specifying the path to the endpoint to check the readiness of the service.
readiness_probe: /health
# How many replicas to manage.
replicas: 2
envs:
MODEL_NAME: meta-llama/Llama-2-7b-chat-hf
HF_TOKEN: # TODO: Fill with your own huggingface token, or use --env to pass.
resources:
accelerators: {L4:1, A10G:1, A10:1, A100:1, A100-80GB:1}
ports:
- 8000
setup: |
conda activate sglang
if [ $? -ne 0 ]; then
conda create -n sglang python=3.10 -y
conda activate sglang
fi
pip list | grep sglang || pip install "sglang[all]"
pip list | grep transformers || pip install transformers==4.37.2
python -c "import huggingface_hub; huggingface_hub.login('${HF_TOKEN}')"
run: |
conda activate sglang
echo 'Starting sglang openai api server...'
export PATH=$PATH:/sbin/
python -m sglang.launch_server --model-path $MODEL_NAME --host 0.0.0.0 --port 8000
llava.yaml
service:
# Specifying the path to the endpoint to check the readiness of the service.
readiness_probe: /health
# How many replicas to manage.
replicas: 2
envs:
MODEL_NAME: liuhaotian/llava-v1.6-vicuna-7b
TOKENIZER_NAME: llava-hf/llava-1.5-7b-hf
resources:
accelerators: {L4:1, A10G:1, A10:1, A100:1, A100-80GB:1}
ports:
- 8000
setup: |
conda activate sglang
if [ $? -ne 0 ]; then
conda create -n sglang python=3.10 -y
conda activate sglang
fi
pip list | grep sglang || pip install "sglang[all]"
pip list | grep transformers || pip install transformers==4.37.2
run: |
conda activate sglang
echo 'Starting sglang openai api server...'
export PATH=$PATH:/sbin/
python -m sglang.launch_server --model-path $MODEL_NAME --tokenizer-path $TOKENIZER_NAME \
--chat-template vicuna_v1.1 --host 0.0.0.0 --port 8000